Matrix AI Assist
Designing a Generative-AI-powered tool that proactively embeds the right information into employee workflows.
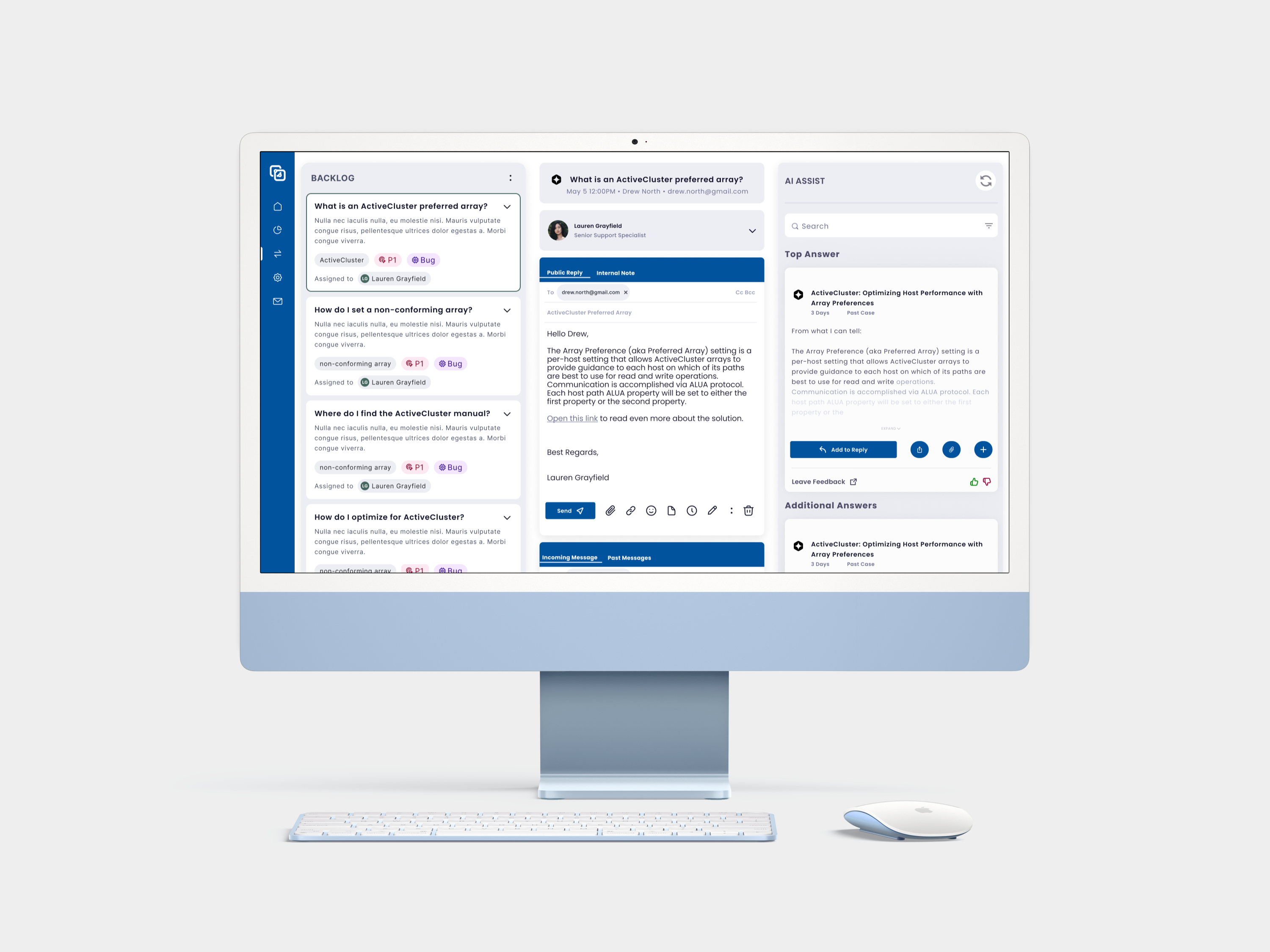
Project Details
Product Design
Primary Practice Area2023
YearLead Designer
My Role
Challenge
About Matrix AI Assist
Overview
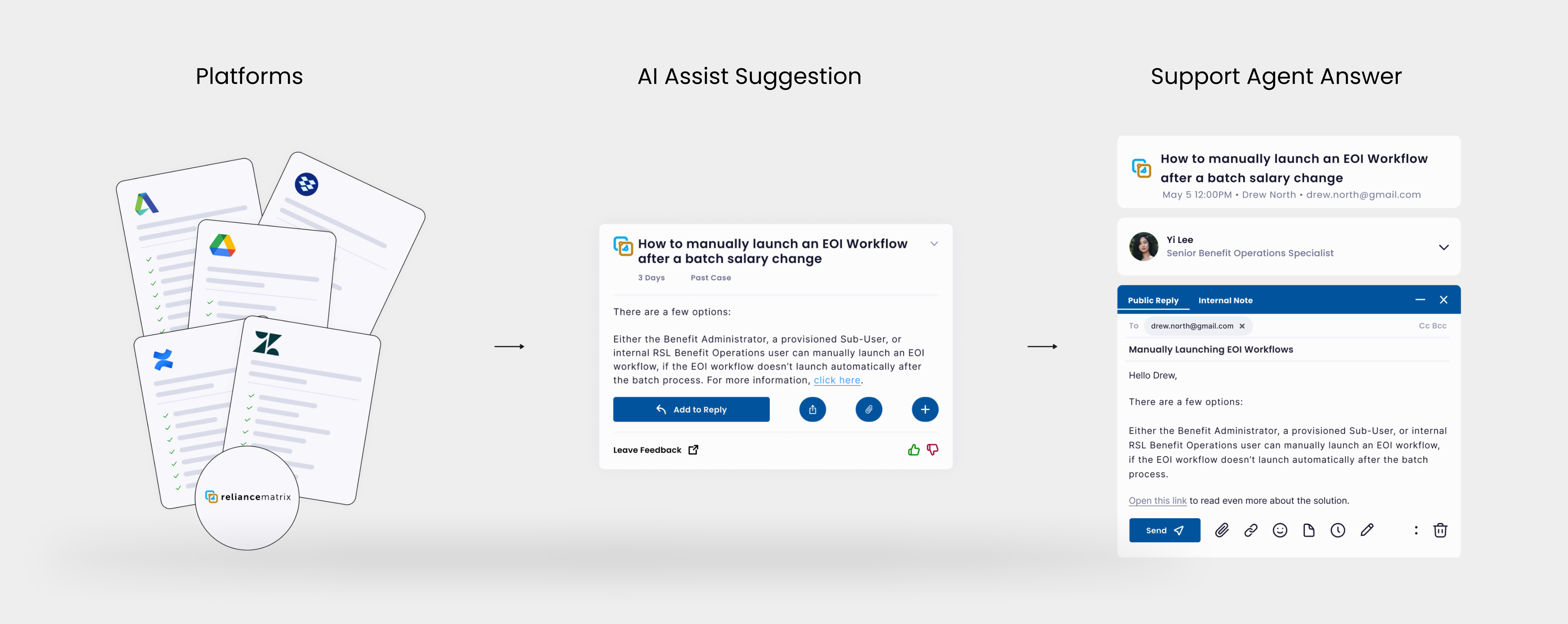
The Design Process
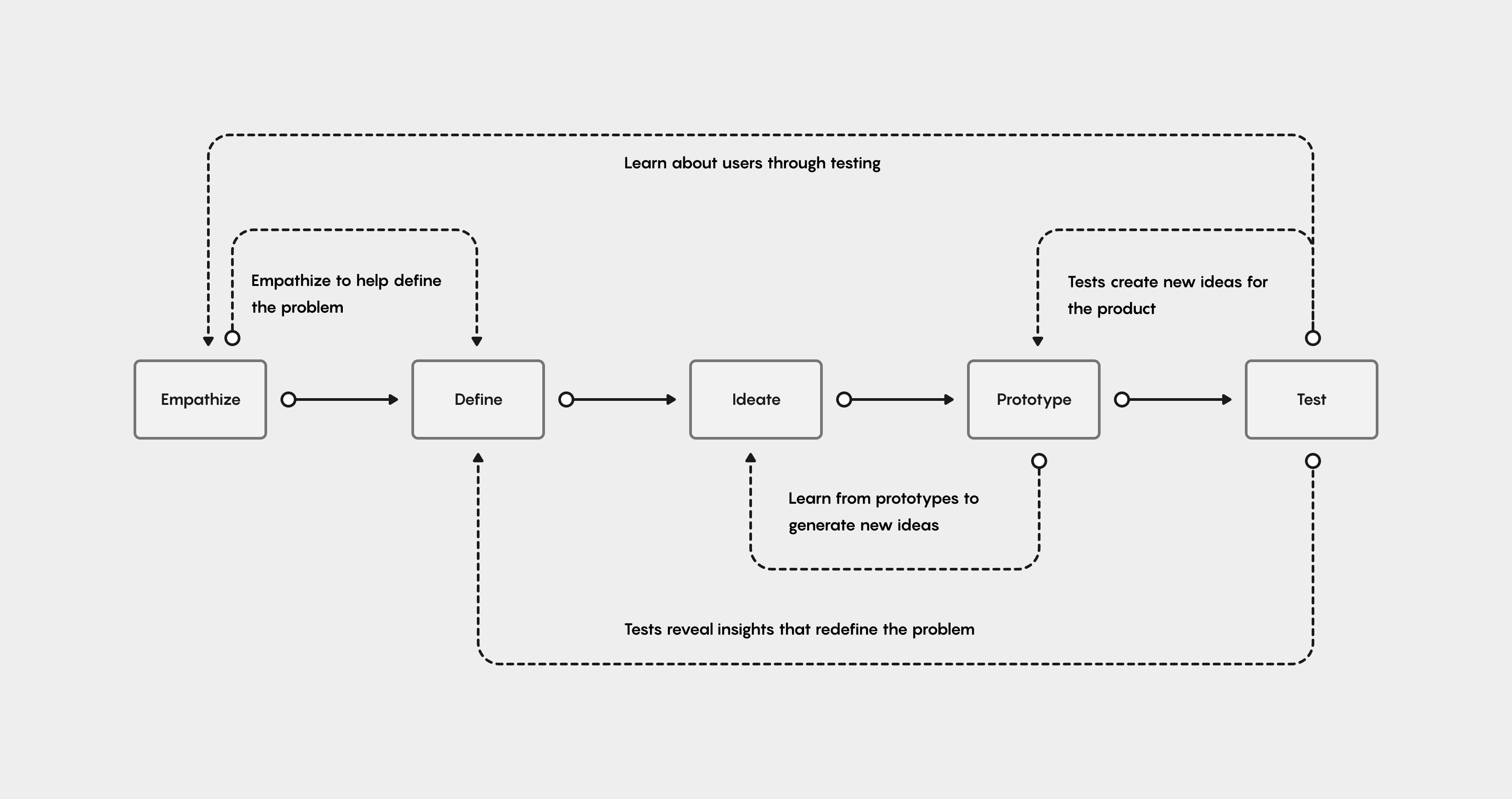
The Users
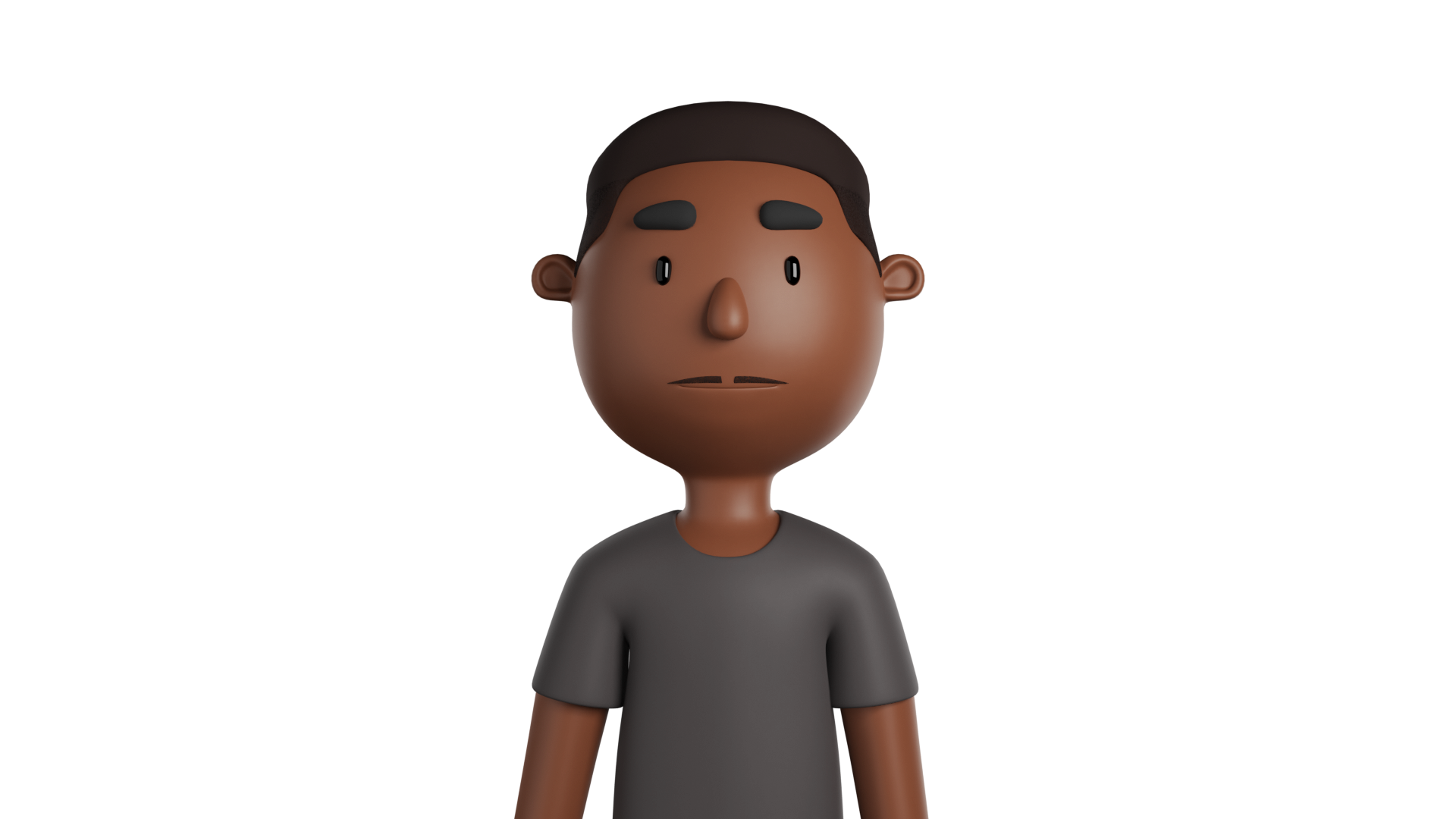
Benefit Ops Support Agents
Benefit Ops Support Agents answer dozens of relatively complex questions per day. On average, they spend between 5 and 15 minutes on each question. These agents tend to be highly empathetic & creative, typically coming from backgrounds in retail or education.
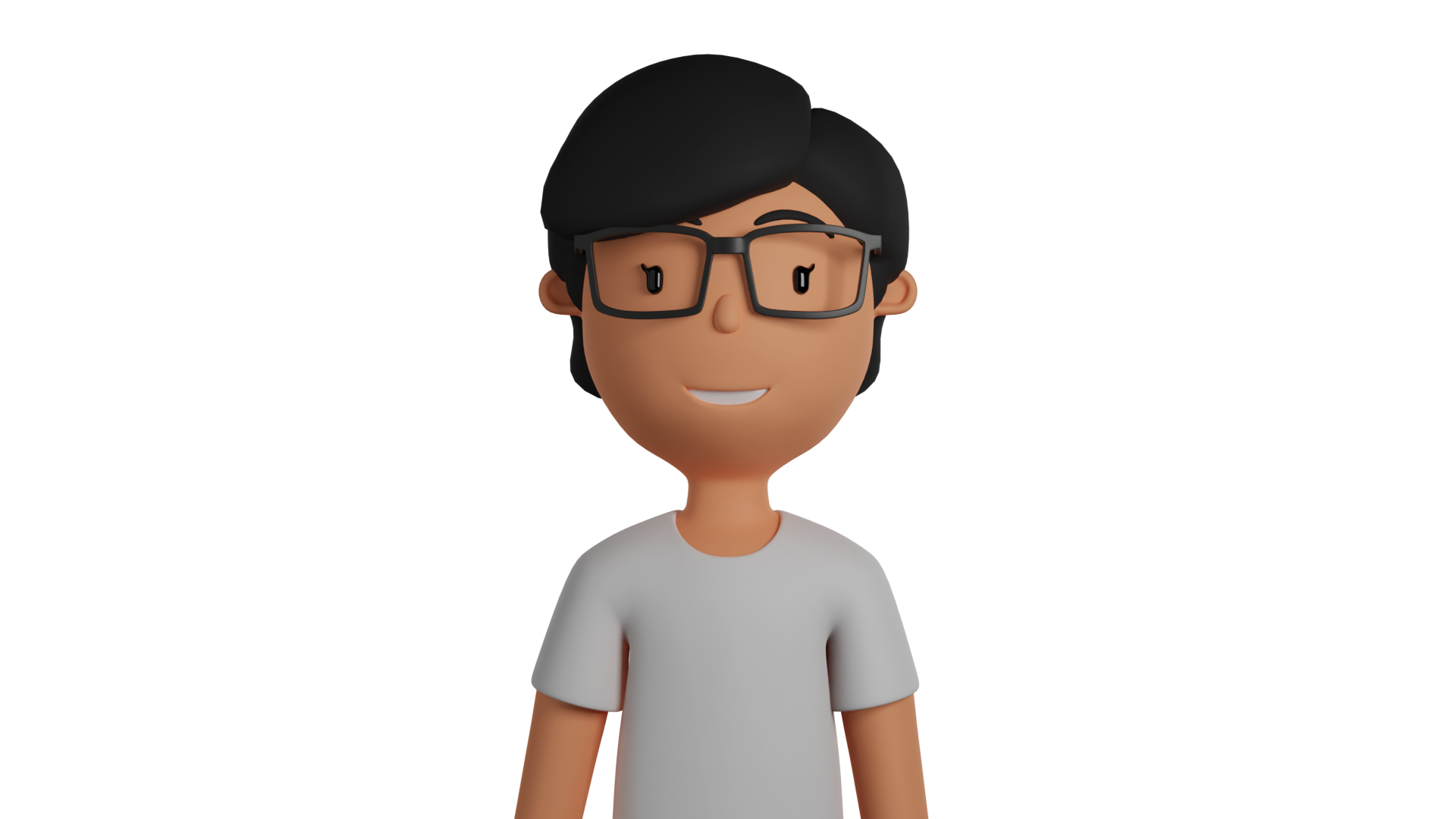
Power Agents
Power Agents are typically Senior Support Agents or Managers. Their typical day is spent onboarding new agents, setting up integrations, triaging problems, training, & tracking insights on impact. They also evaluate potential opportunities to fill in gaps across data stores.
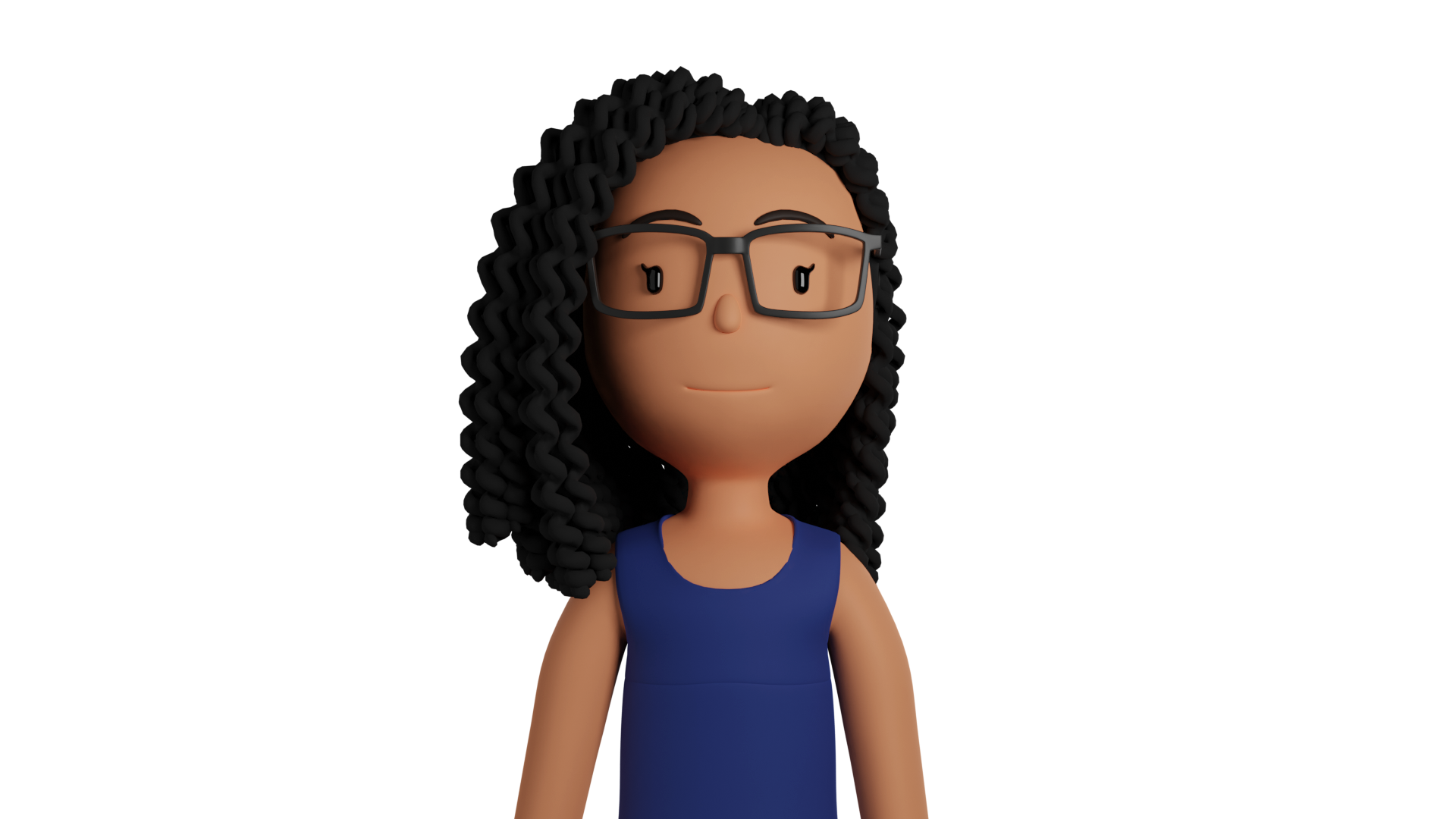
Benefit Administrators
Benefits Administrators manage an organization's employee benefit programs, such as health insurance, supplemental benefits, and retirement plans. They ensure: benefits are implemented efficiently, employees understand them, and that they comply with relevant legislation.
User Research

I start off every design project with a practical research canvas to establish a clear purpose, set clear goals and measures of evaluation, to help define the problem space, to clearly identify initial assumptions, to lay-out hard deliverables, and to choose the appropriate research methods.
For the initial research on Matrix AI Assist:
-I ran initial surveys to gain initial discovery insights about our user's needs and frictions points, in how they solve customer support tickets. This helped me understand the what and where behind the users pain points.
-I used contextual inquiries and user interviews to help contextualize the 'why' and 'how' behind the usability issues, and to uncover additional user pain points.
-I led interviews with internal stakeholders to understand and align on the business objectives and product metrics.
-Later on, I leveraged a benchmarking usability test of the MVP to evaluate the initial usability, and establish a basis of comparison for all subsequent iterations.
Measures of Evaluation
To help with initial alignment between the design team and the business, I leveraged a set of existing product metrics and KPI's, initially set by the business.
I tested these metrics during the usability tests, alongside traditional usability metrics like time on task, task completion rate, and error rate.
Agent Velocity
How long it takes agents to solve a ticket.Agent Accuracy
The accuracy of an agent’s given answer.AI Accuracy
The accuracy of the top suggestion given by the AI, to the agent.Feedback Velocity
How long it takes an agent to provide feedback on an AI suggestion.Feedback Accuracy
Quality of the feedback given by an agent.
Synthesizing Research Data

Once initial research was complete, I used affinity analysis to uncover key themes I could turn into actionable ideation points and formalized design challenges.
Research Artifacts
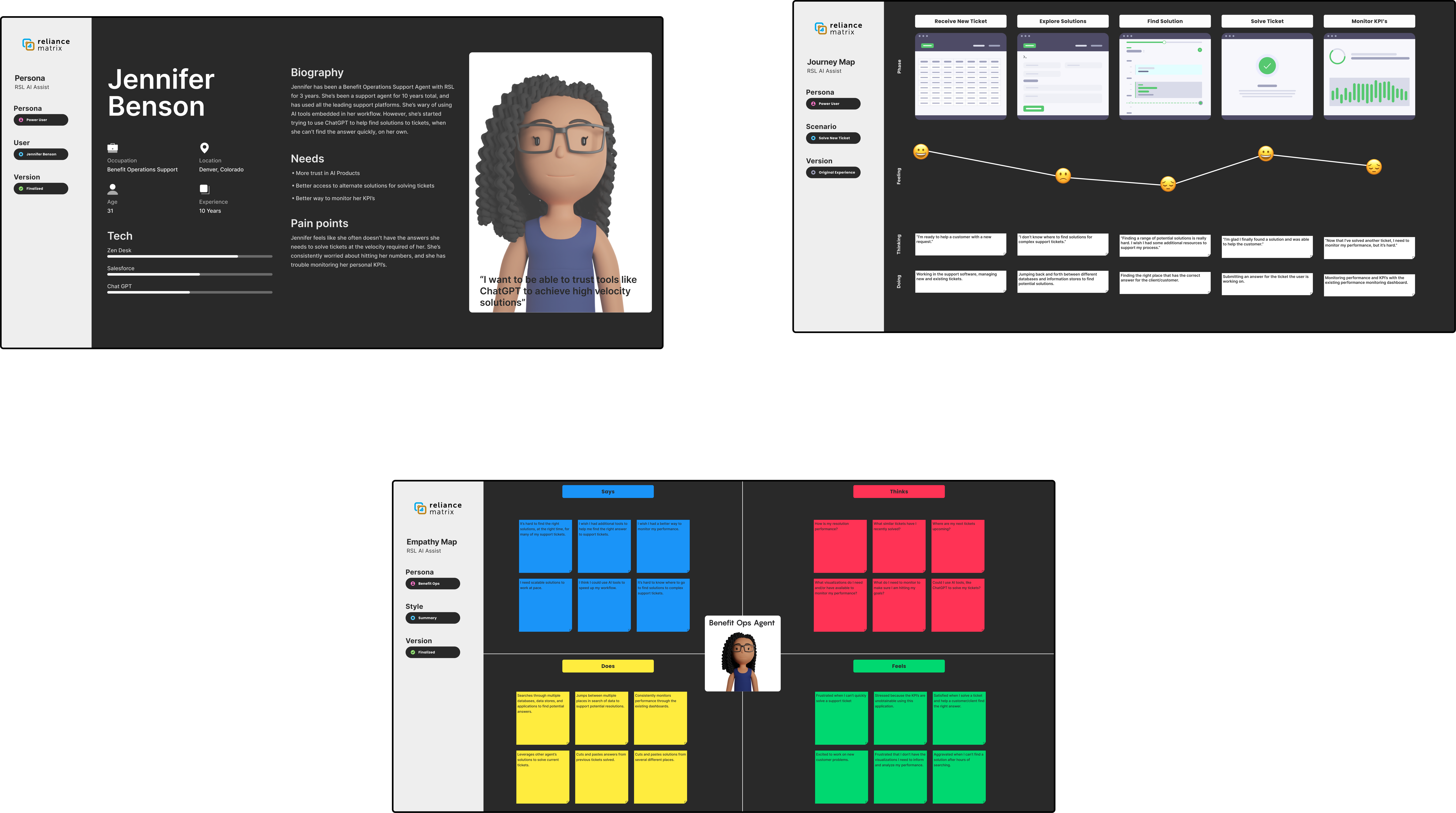
I created personas based on real users, journey maps, and empathy maps to bring-life to the key research themes.
Using high fidelity research assets helps me set a high bar for designscraft, and helps maintain stakeholders interest when used in the context of reports and presentations.
Key Problems To Solve
How might we reduce load on support agents?
How might we foster feedback to train the GenAI Model?
How might we design for inflexibly small surfaces?
How might we ensure users are confident in AI-generated answers?
How might we design for sales growth?
Assessing The MVP
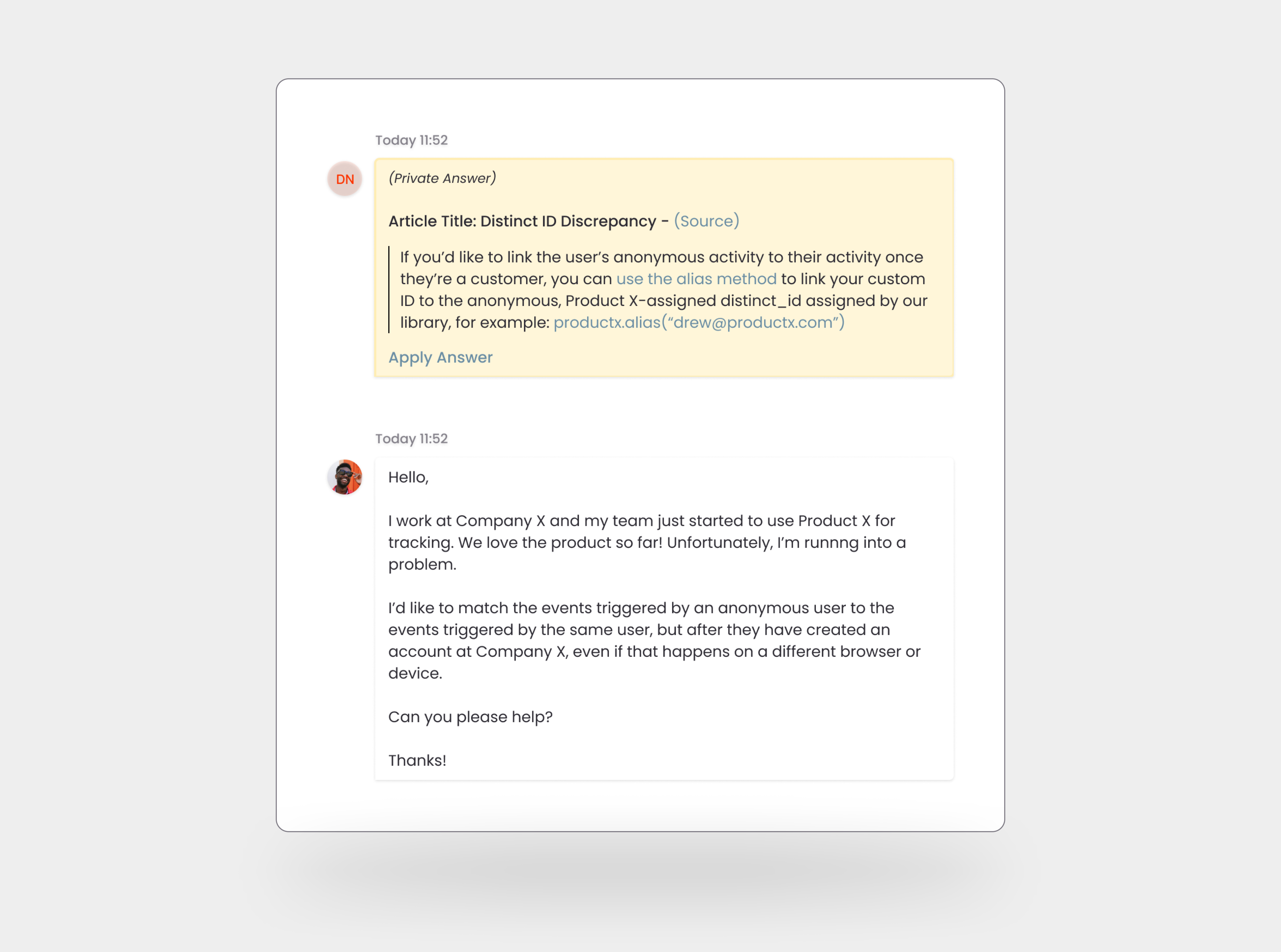
The POC version of the product was a simple text interface. The product, AI Assist, analyzed new tickets/cases, and then left a simple, private comment (only visible to a support agent) on the ticket with a recommended customer resolution for the agent.
Designing for Inflexibly Small Surfaces

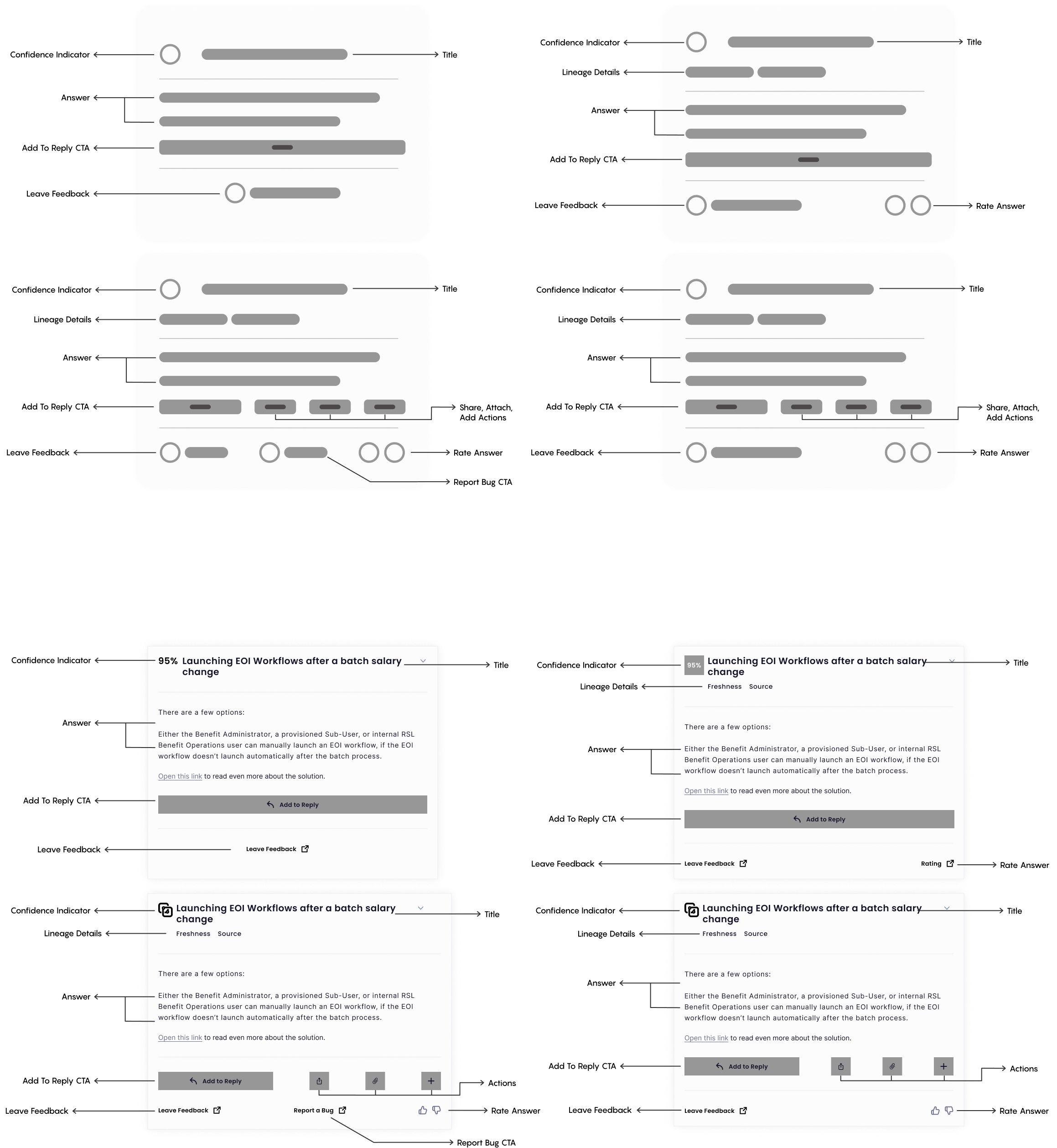
The first challenge was to design for inflexibly small surfaces.
Since there was a lot of data that I needed to fit into the small surface of the answer card, I needed to assess and prioritize the information I'd need to present to the users.
I used the initial user interviews to uncover the information the users needed to make informed decisions about potential answers, and used stakeholder interviews to understand what items the business needed to have represented in the answer card.
Must have's for the users were clear topic title, clear answer, and the ability to add the reply to a customer message. Nice to have's for the users was lineage information like data freshness and data source.
The business needed a mechanism to submit feedback and rate the answer, to further train the model, and bug reporting was also an additional nice-to-have.
From my conversations with users, I also knew we needed to bake in a confidence indicator that would ease the user's reservations about accepting an AI-generated answer.
I started with paper wireframes and transitioned to low-fi and mid-fi wireframes. Then tested 4 separate variations with the users in order to validate the final direction.

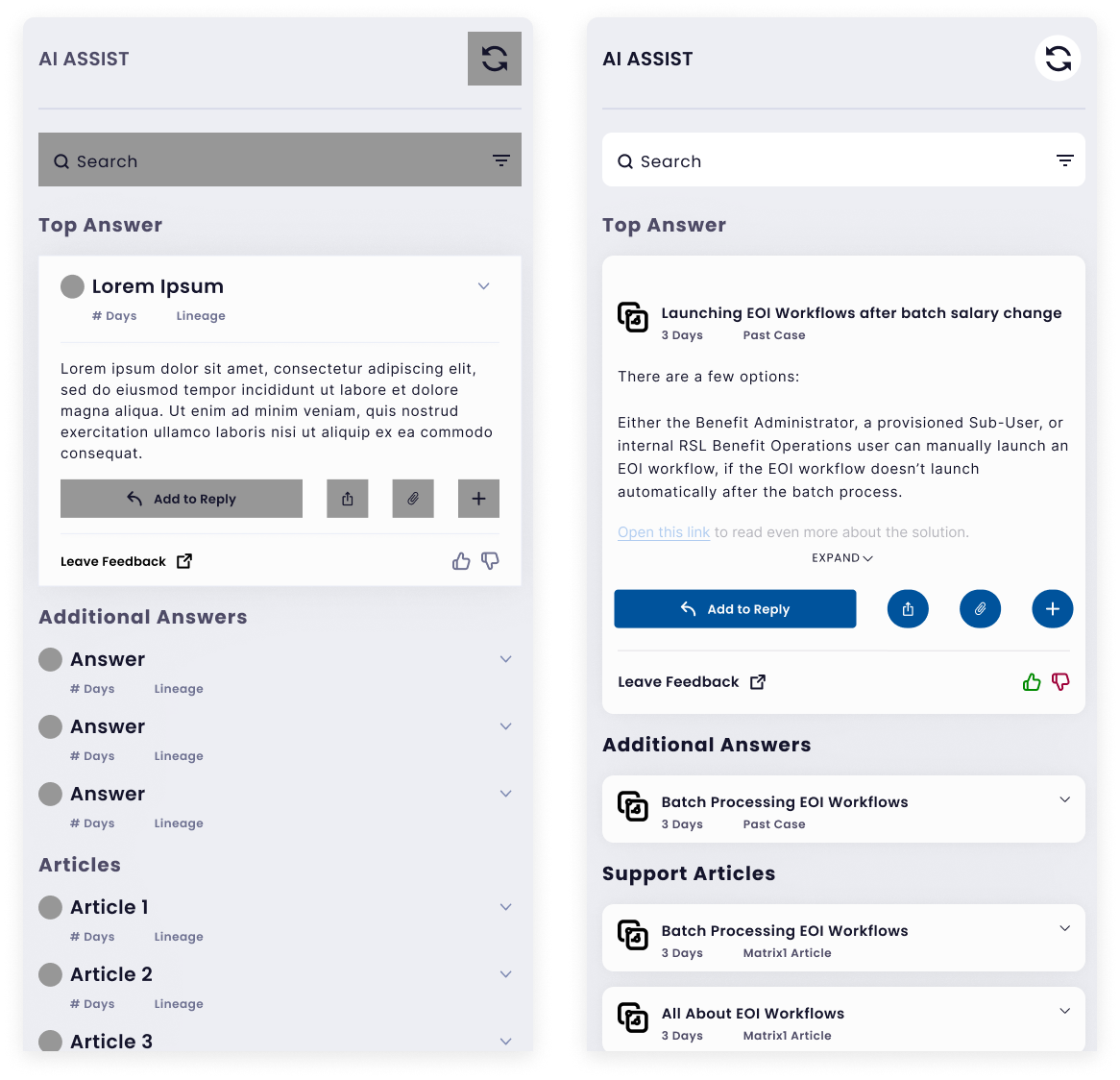
Once I had the primary card design finalized and validated, I could start designing the rest of the AI Assist module. Again, starting with paper wirframes and transitioning to low and mid-fi wireframes to test with the users.
Reducing Load On Support Agents
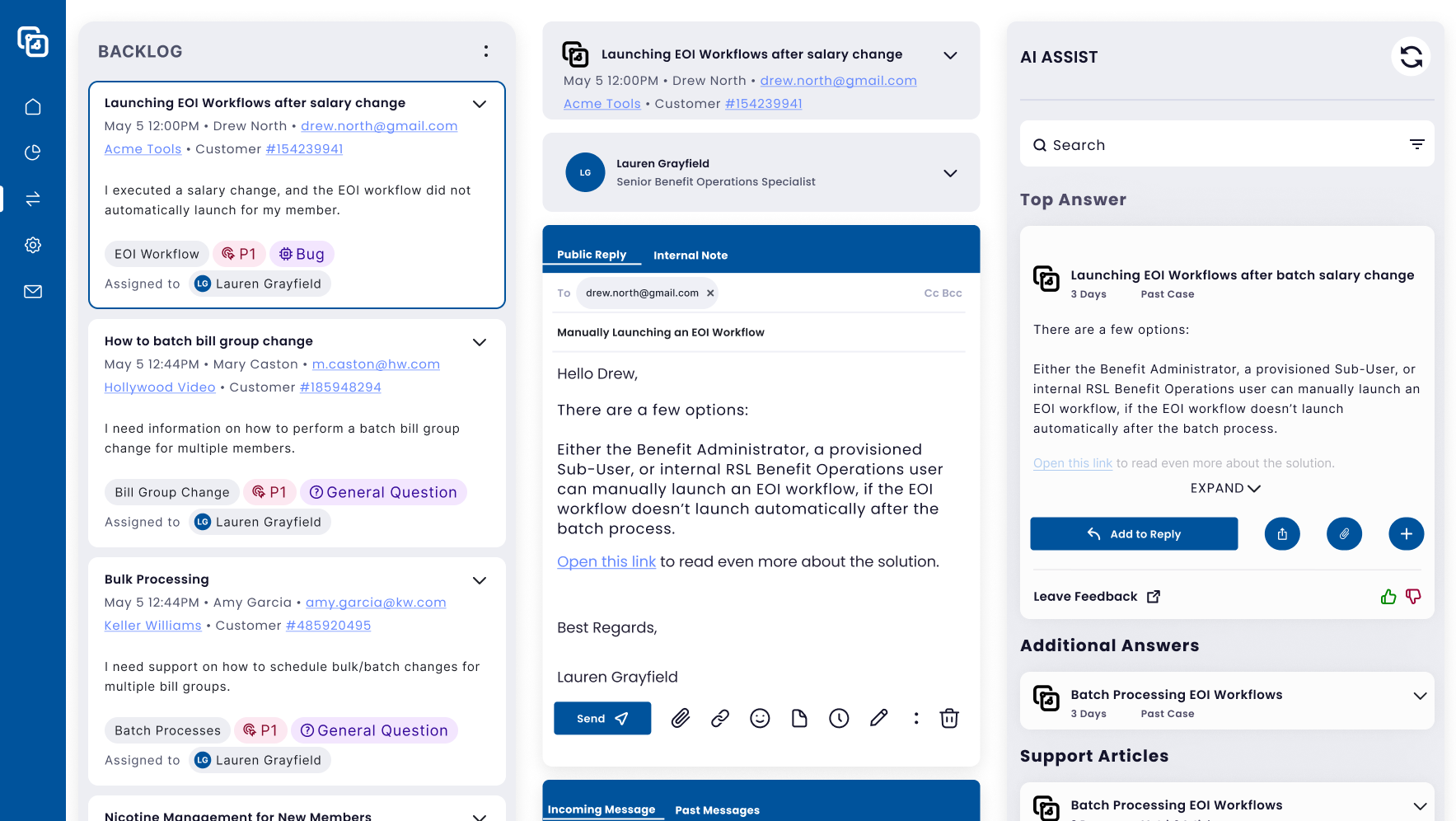
Here's the final AI Assist module design, seen in the context of Reliance Matrix's proprietary internal CRM.
This leads into the next design challenge which was to reduce load on support agents.
The core benefit of AI Assist is that it works silently in the background, as agents go about their normal work. To make it seamless for agents to go between their current ticket & AI Assist's suggestions, reducing their overall workload, I implemented several outcome-driving design features.
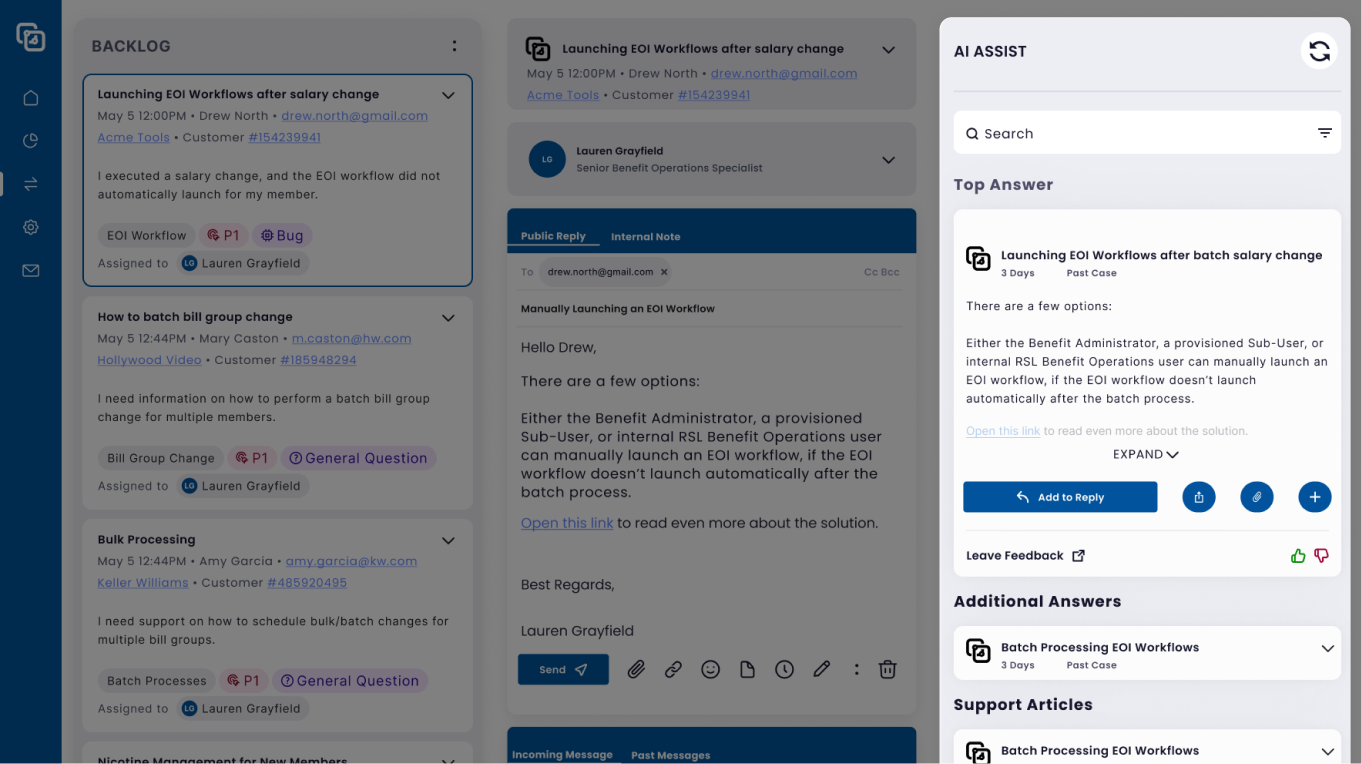
I placed the AI Assist module near the ticket, and high up on the page, to the right of the ticket respones. The top suggestion is automatically queued up for the support agent. With one button click to add the solution to the reply. The user can also easily share the answer with other agents, attach files, or ammend the top answer with additional context.
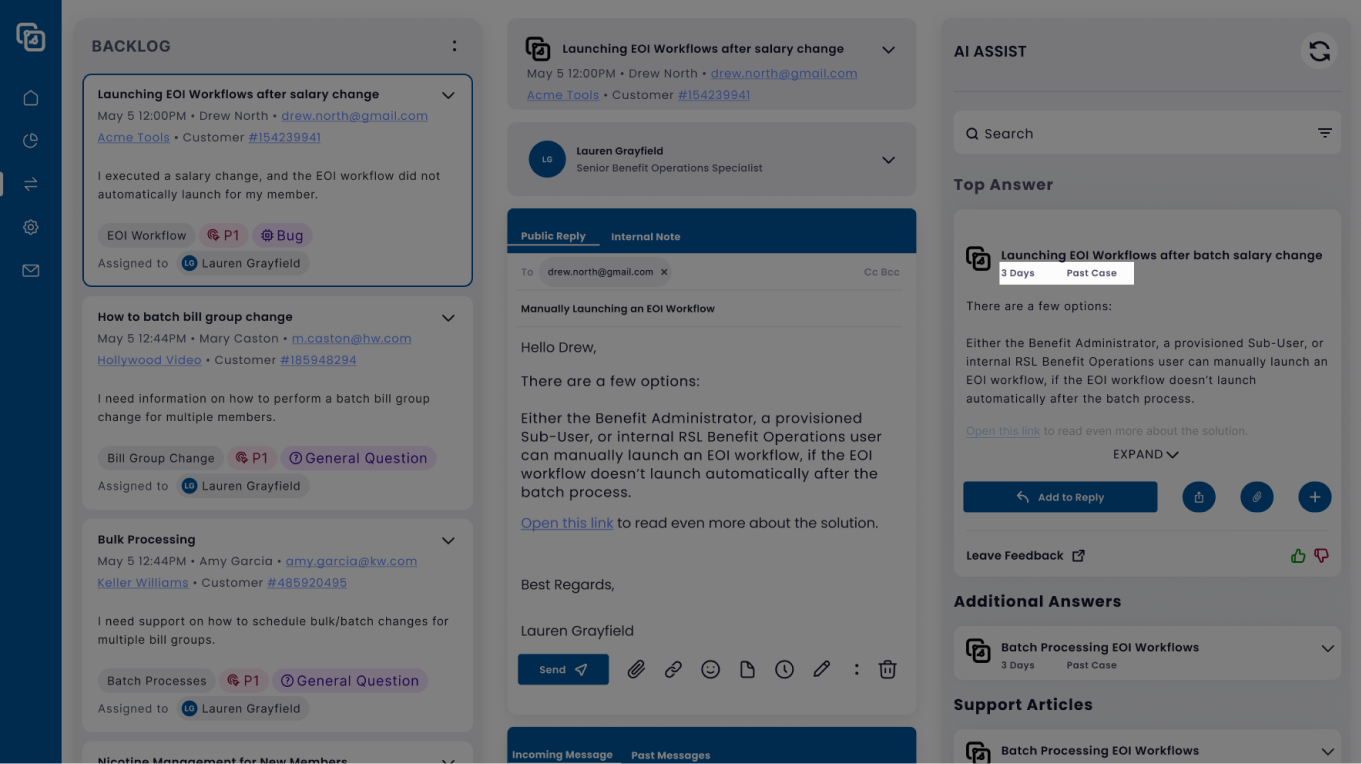
The extra details provided under the answer title allow the user to see the freshness of the answer, and the lineage of where the answer originated.
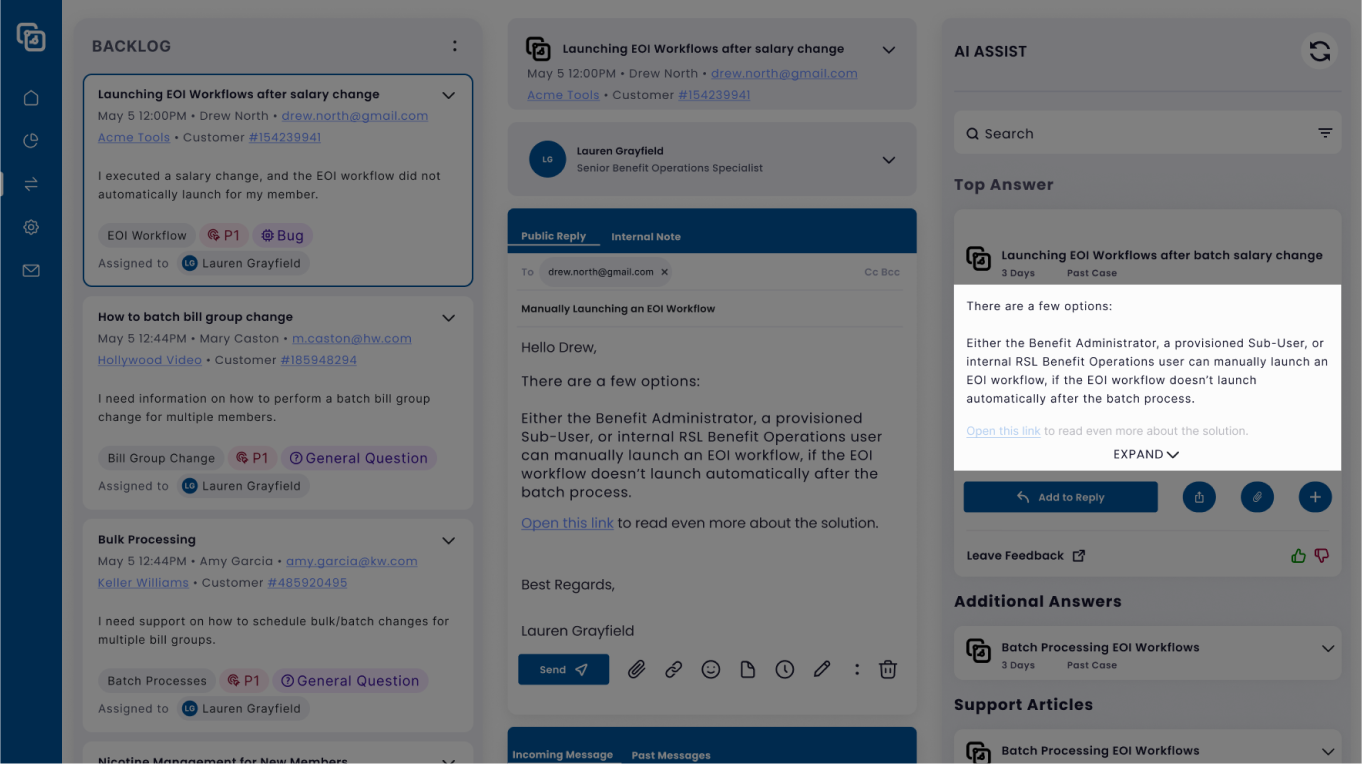
Since I was working with a small surface I needed to make the text portion expandable and collapsable to ensure the support agent can quickly preview the top answer, as well as easily see and read the full answer.
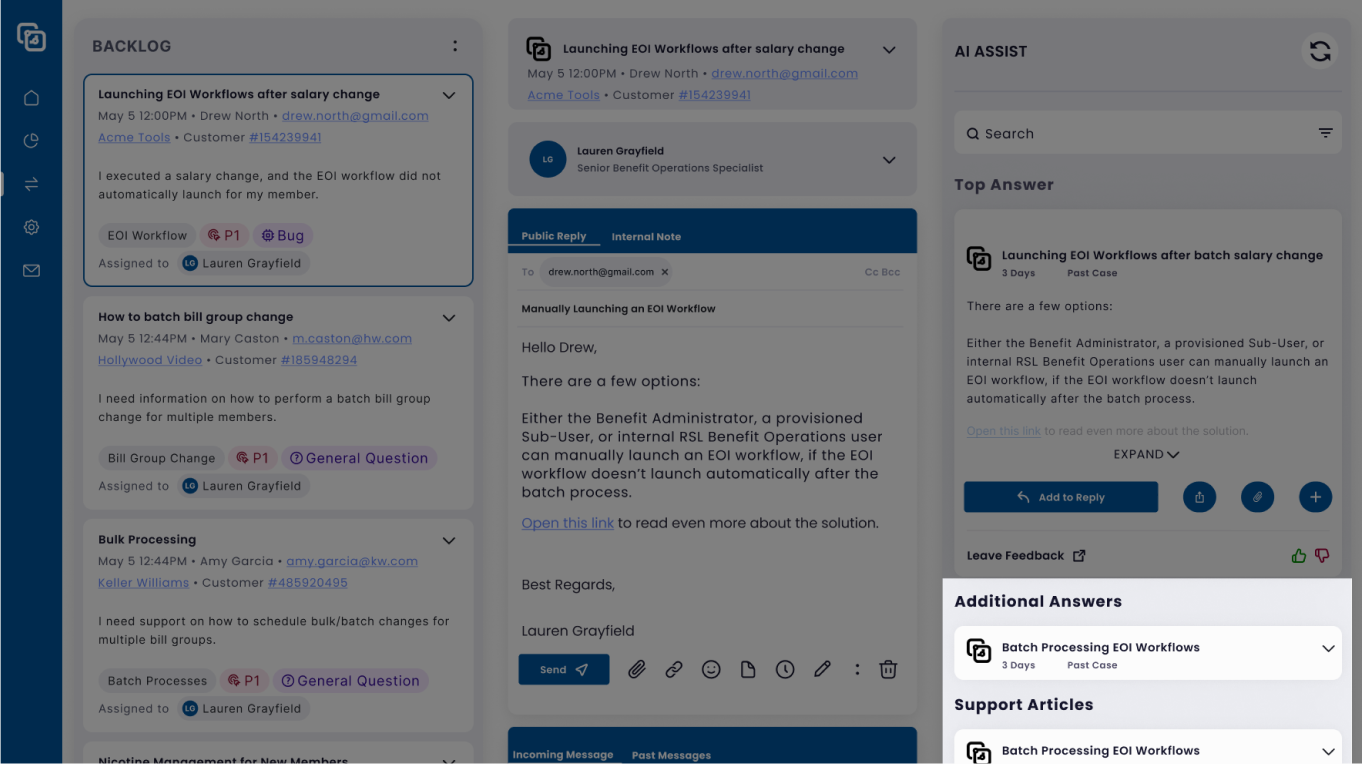
Under the Top Answer module the user has the ability to explore additional answers and curated articles, if the top answer doesn't work.
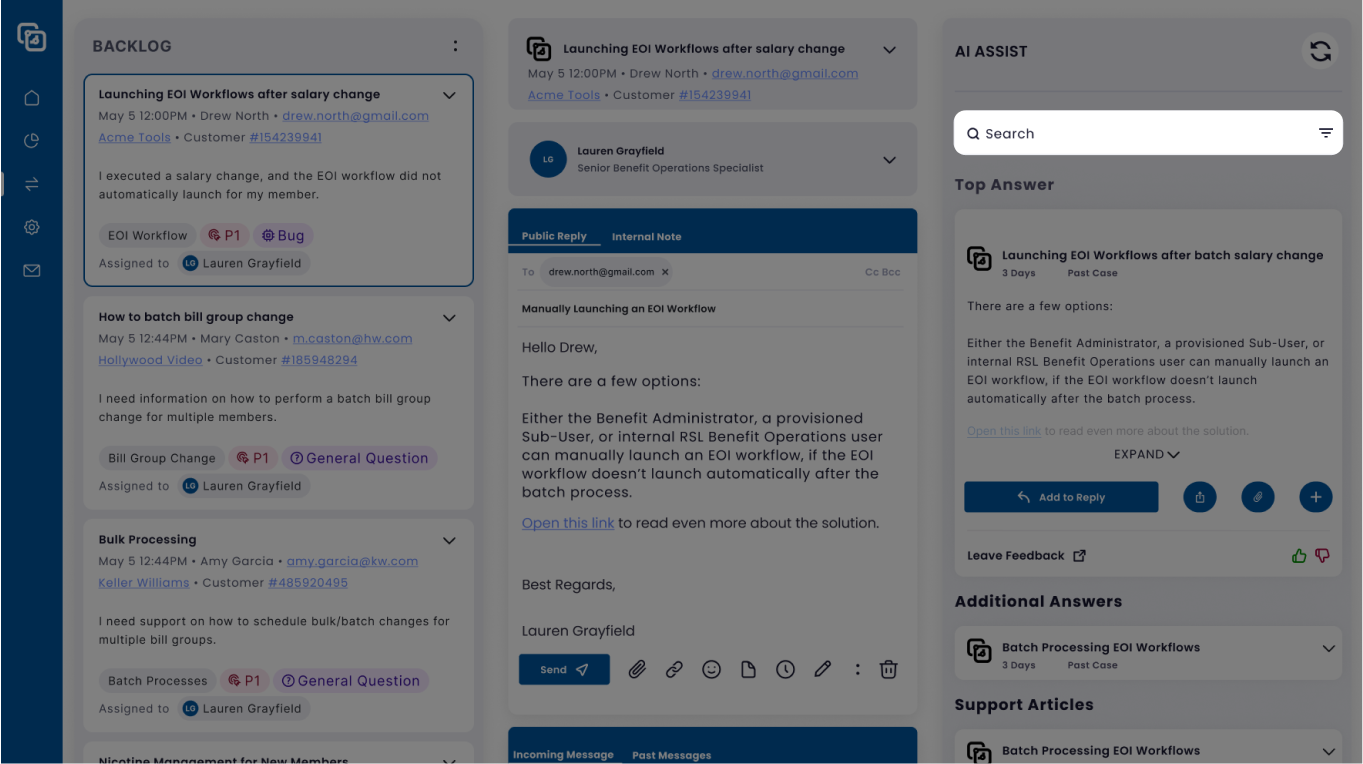
In case none of the additional answers or articles suffice, the agent has comprehensive search and filtering capabilities to support the search for additional answers.
Enhancing User Confidence
Iteration 1
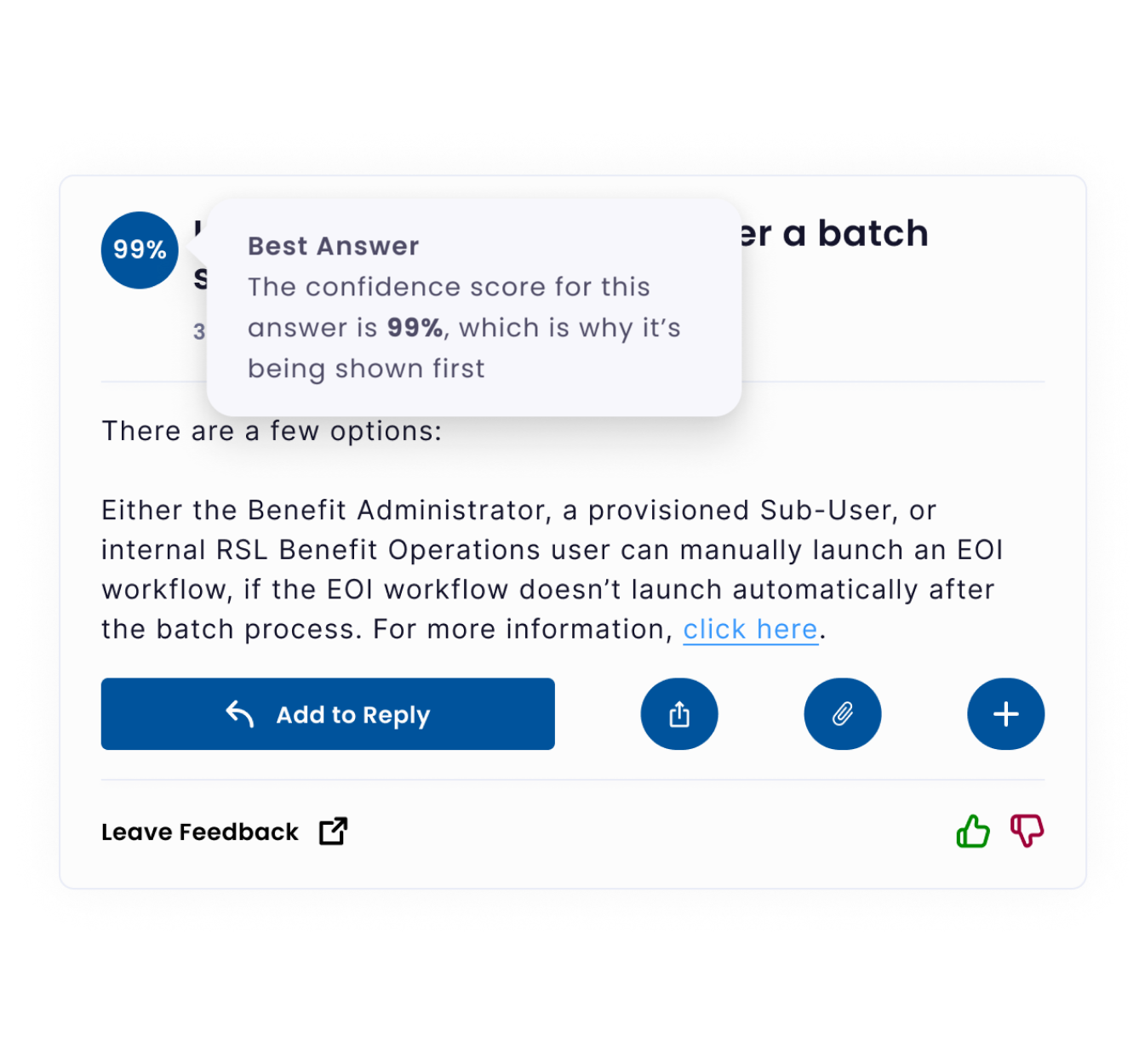
Iteration 2
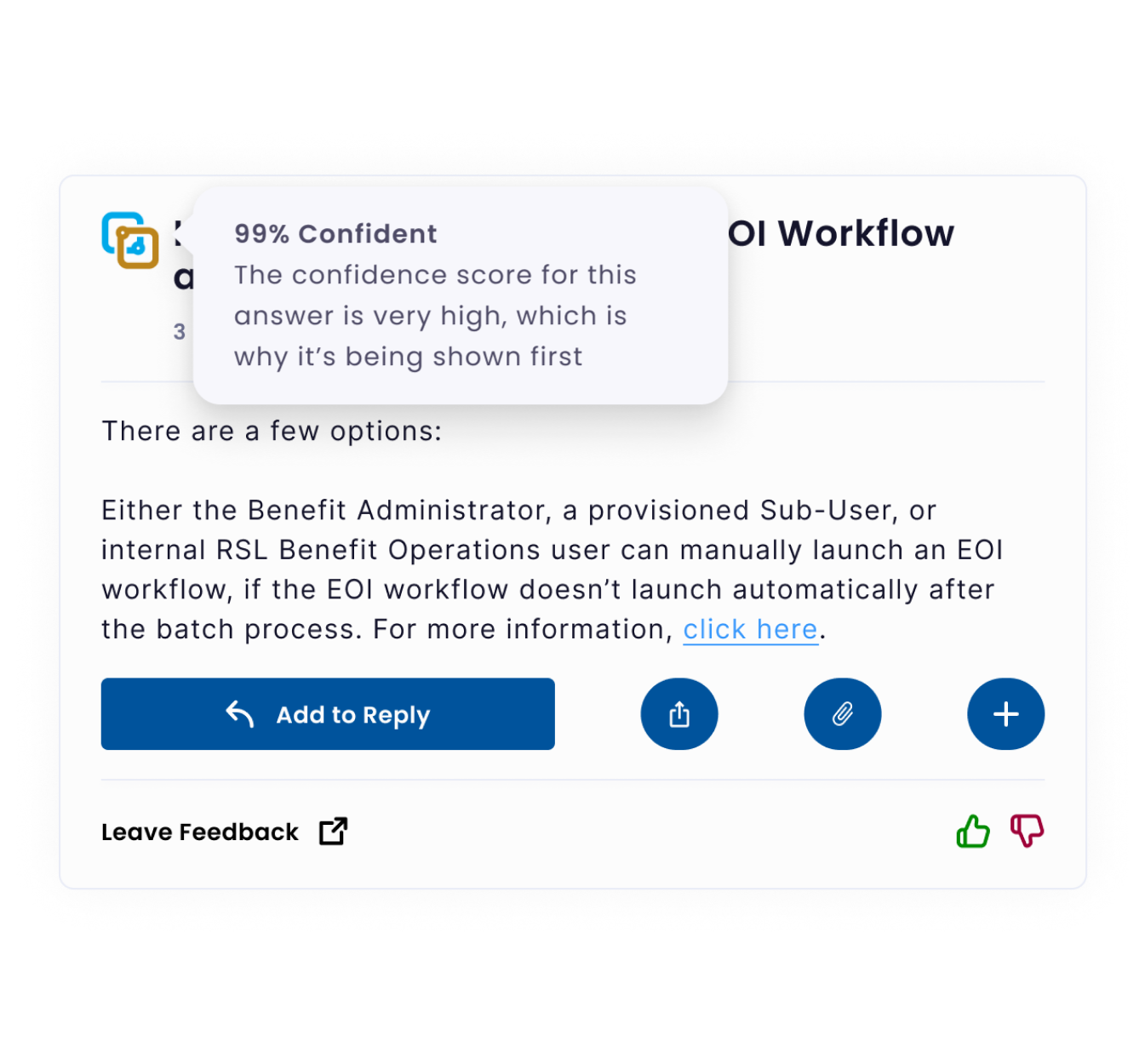
Iteration 3
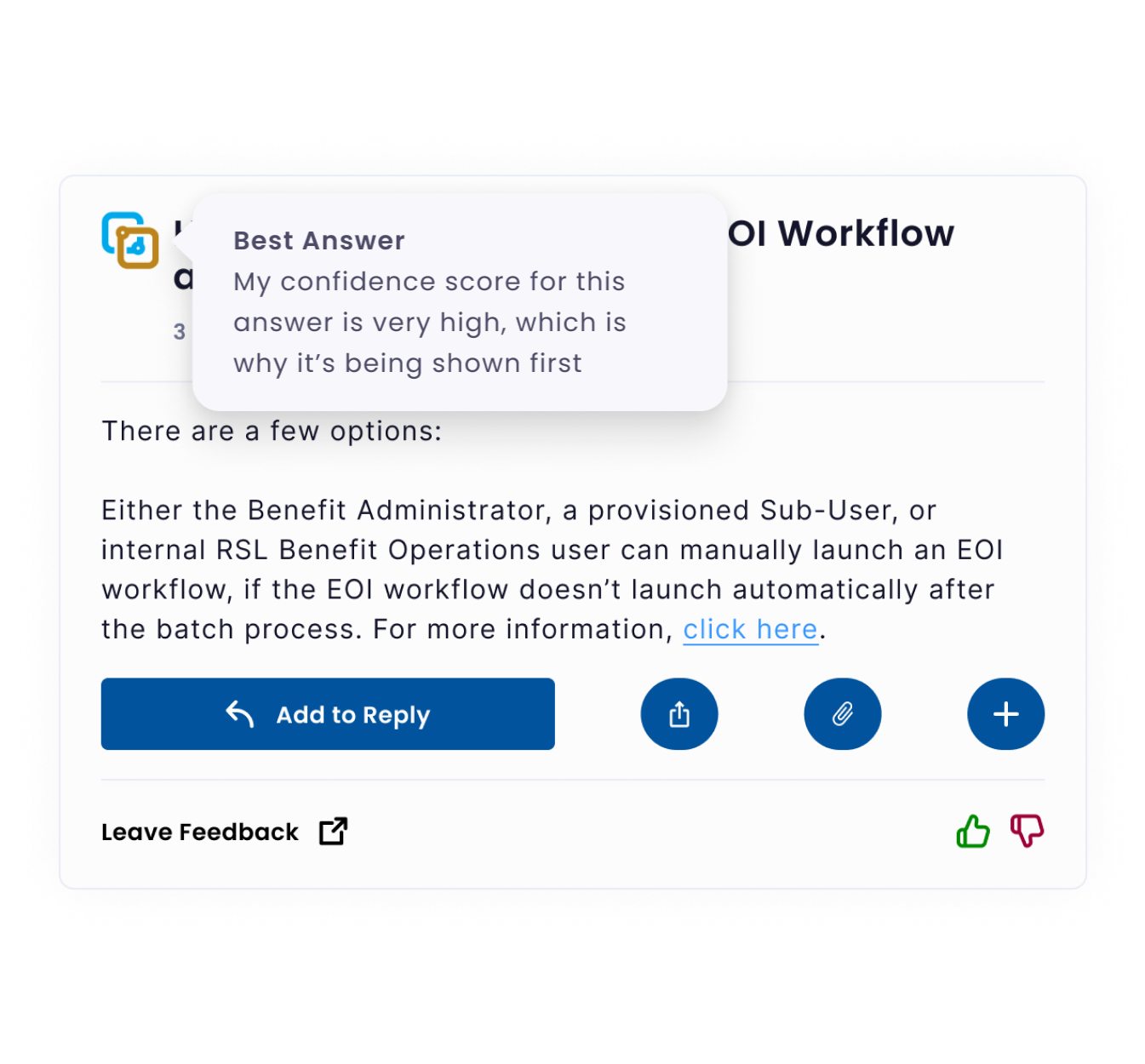
Iteration 4 (Final)
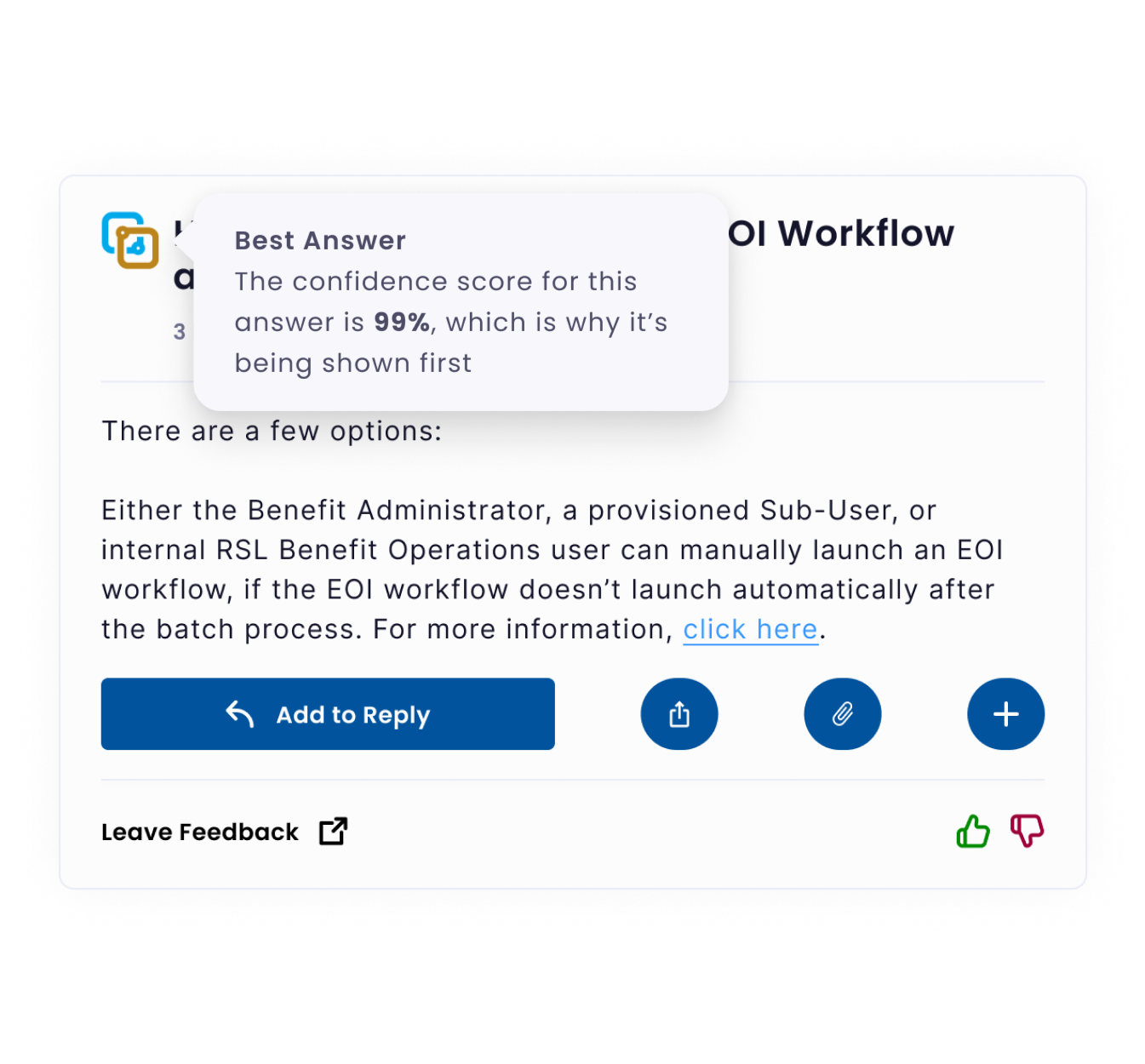
The next design challenge, a unique problem I uncovered in the user interviews, was the support agent user's general distrust in generative AI solutions.
I needed to design a quick and easy way to get users over the trust barrier. The best solution was to implement an AI confidence indicator. I designed four different versions of the confidence indicator.
I tested both qualitative and quantitative metrics to display answer confidence, and tested different types of interactions to display additional confidence indicator context.
Upon testing with the users, they preferred a hover interaction with tooltip to see a quantitative measure of the answer accuracy.
Making the Product Smarter with User Feedback
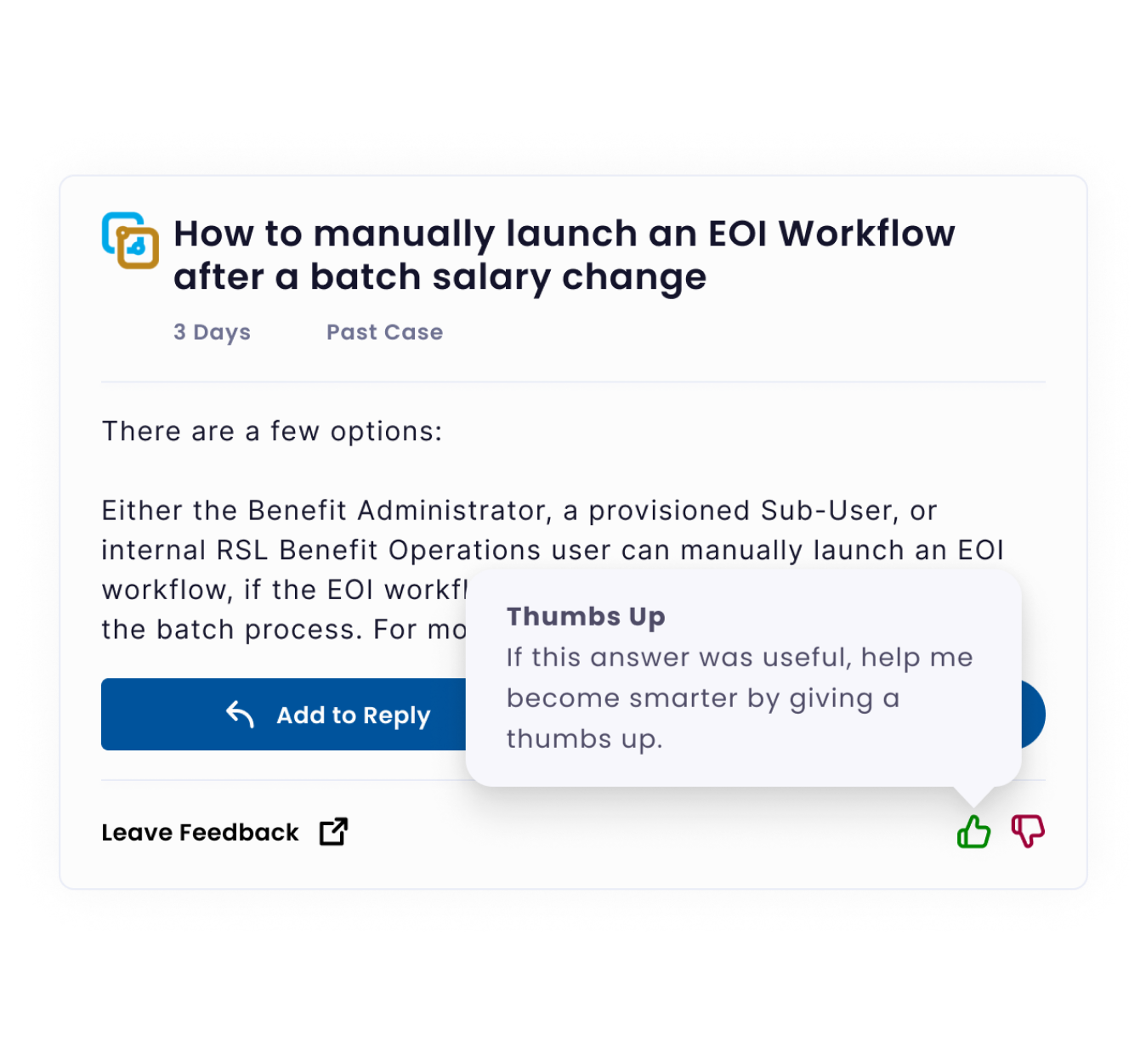
The next design challenge was to incorporate a lightweight mechanism for user-driven AI model training.
The product's accuracy is driven by the ability to capture user feedback in real-time.
Support agents are given the opportunity to further train the model by giving thumbs-up or thumbs-down feedback to each suggestion.
Additionally, the support agents can leave detailed feedback about the answer, and even add additional context or answers that can further train the AI model.
I also worked with the product team to design training documentation that incentivizes users to leave feedback, demonstrating to the users how the user feedback process help the product get better, and how it translates into better answers to complex problems.
Designing For Sales Growth
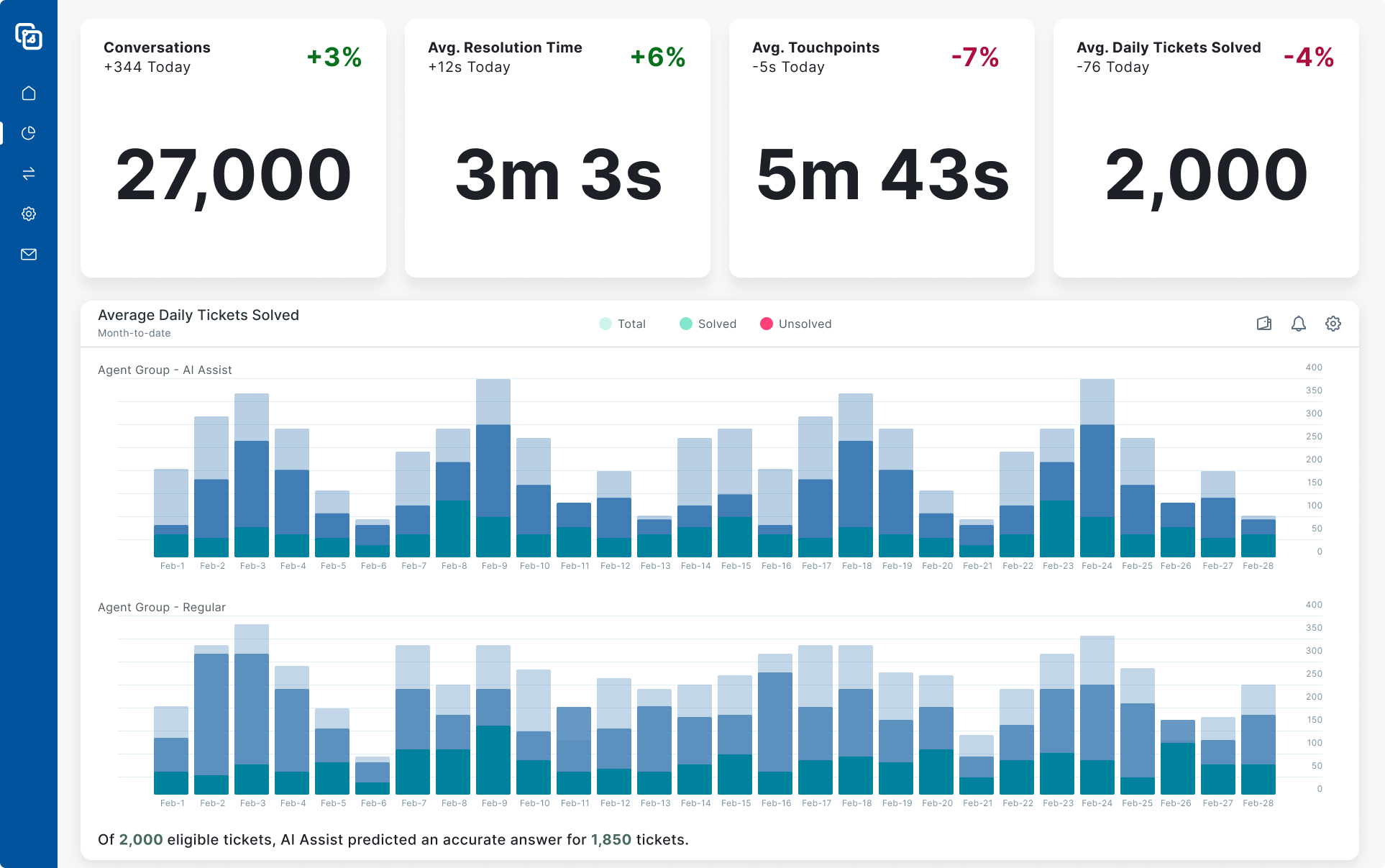
The final major design challenge I want to highlight is the admin dashboard. Administrators and power agents are especially interested in understanding and demonstrating return on investment. I designed an efficient admin dashboard that features an A/B test that showcases the performance of AI-Assist-supported agents, compared to those not using AI Assist. This built-in validation feeds leaders the data they need to advocate for keeping AI Assist as a partner.
Integrating The Design System
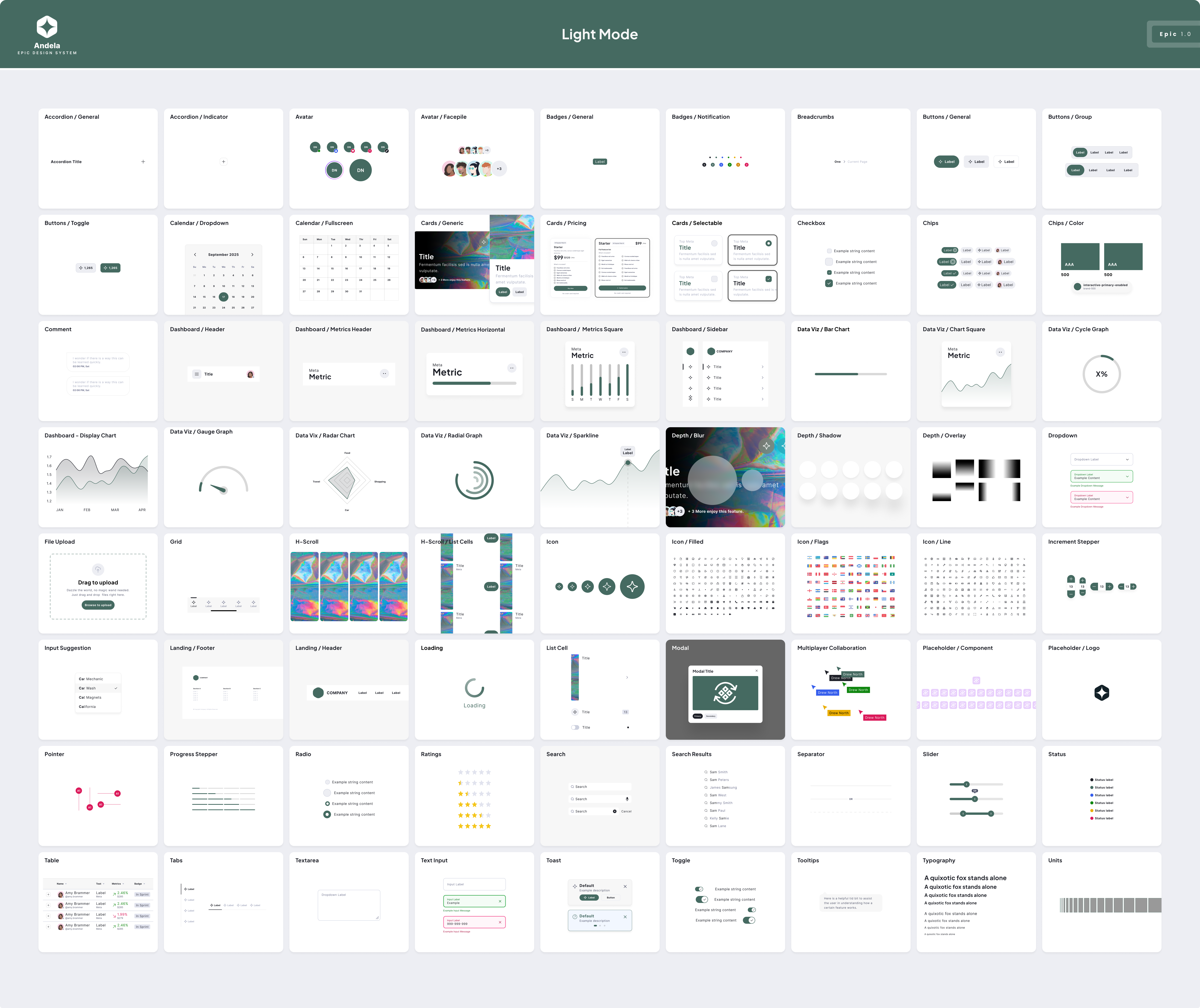
The final UI kit is spun-off from Andela Epic Design System. It's robust and user-friendly, ensuring that the product design team is fully empowered to drive the product's future. With this full UI Kit in-hand, the design team is set up for long-term success, continuing to build, ship, and scale products that meet the same high quality bar.